Premiers pas
Remarque : J’ai écris ce tuto il y a très longtemps, et je ne l’ai jamais publié… mais il peut servir alors je le mets là.
1. Installer Python
Pour commencer, nous allons installer le minimum vital, installer uniquement des outils basiques. Nous verrons plus tard qu’il est possible d’utiliser des outils plus performants, et plus pratiques, mais pour faire ses premiers pas en programmation, c’est un peu compliqué.
Je suppose que vous êtes sous Windows, et mes exemples utiliseront Windows 7.
Nous allons donc commencer par installer Python : c’est bien le minimum nécessaire pour utiliser ce langage de programmation, la librairie standard, l’interpréteur. Nous allons donc pour commencer sur la page www.python.org/download/. Il y a plein de versions disponibles :
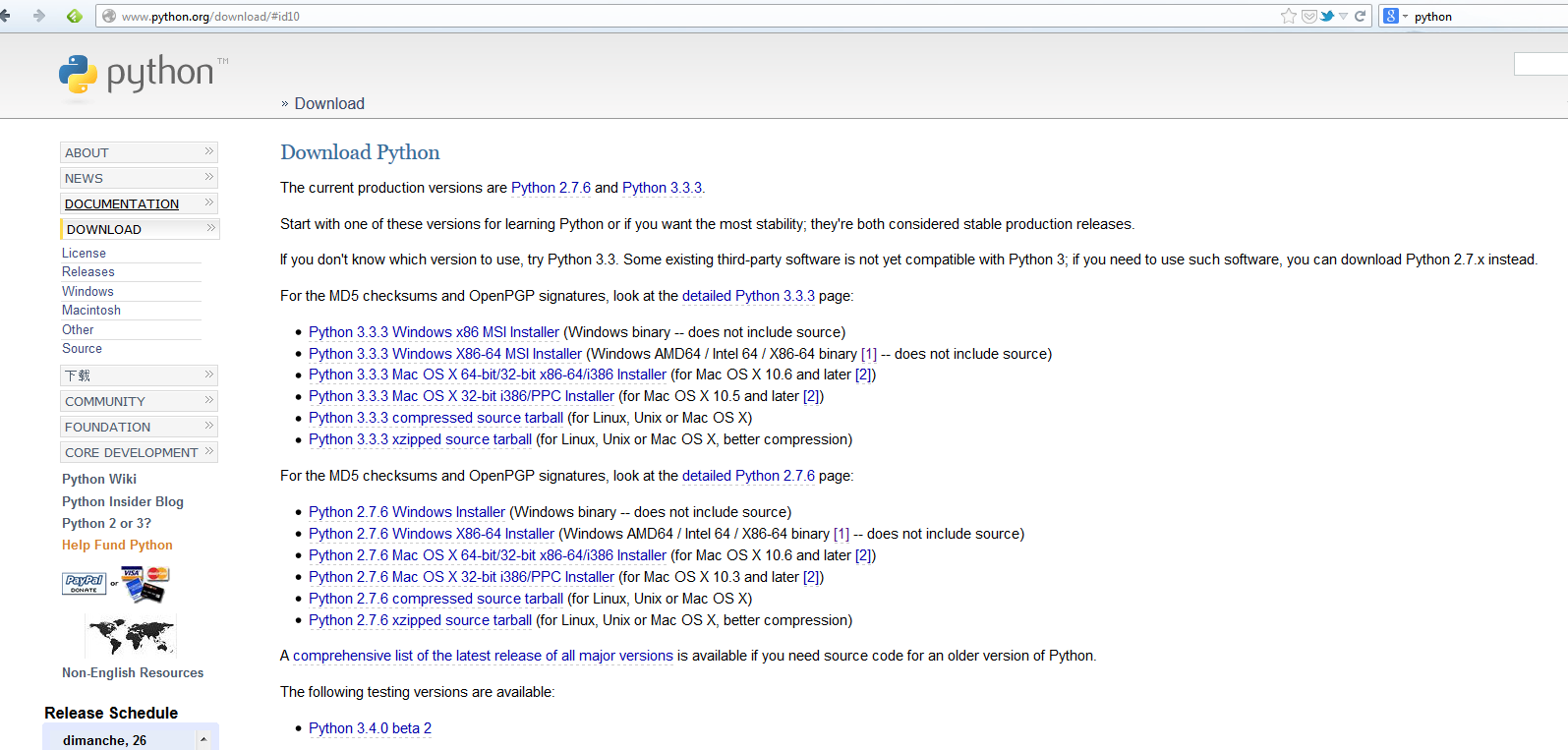
Je vous conseille de prendre la dernière version (3.3.3 au moment où j’écris), avec un installeur MSI (pour Windows, donc) 64bits (si vous n’êtes pas sûr d’avoir une machine 64bits, prenez la version X86 tout court).
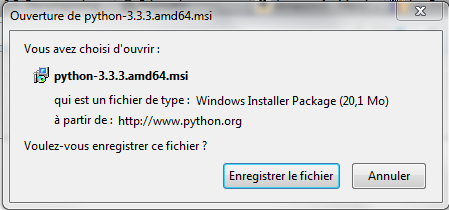
Cliquez sur le lien, puis sur enregistrer.
Exécutez l’installeur en double-cliquant sur le fichier python-3.3.3.amd64.msi
.
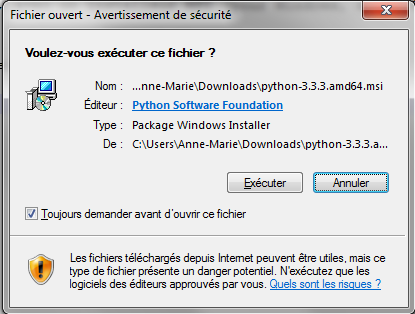
Windows demande confirmation avant d’éxécuter, par sécurité : on fait confiance à la Python Software Foundation, et le fichier a été téléchargé directement depuis www.python.org, donc on peut l’éxécuter en toute confiance : cliquez sur
Exécuter
.
Windows demandera une seconde fois de confirmer après la configuration de l’installation. Pour l’instant, il n’y a qu’à suivre les instruction d’installations :
- installer pour tous les utilisateurs ou non ? Choisissez seulement votre utilisateur si vous n’êtes pas sur votre propre ordinateur.
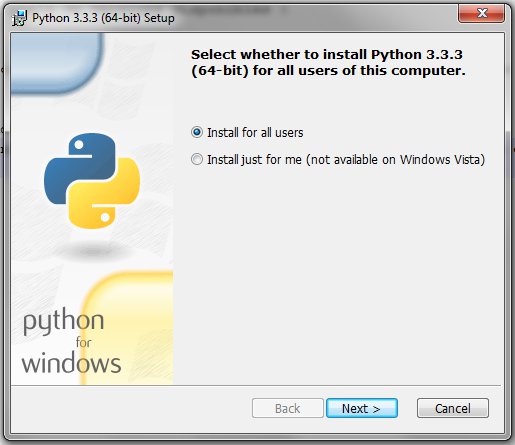
- emplacement par défaut : c’est le répertoire où l’installeur va copier tous les fichiers nécessaires au fonctionnement de Python. Pas besoin de changer.
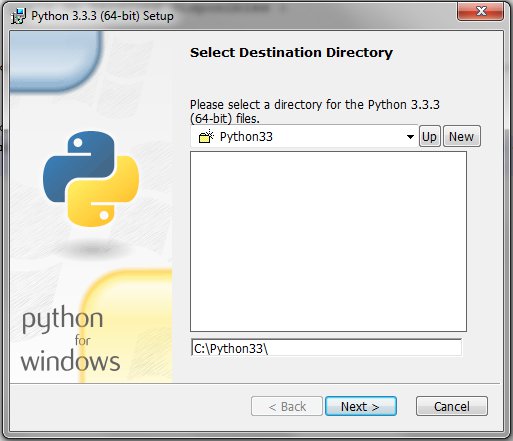
- Personnalisation de l’installation : cocher en plus la seule option qui n’est pas mise par défaut, pour ajouter python.exe à votre path.
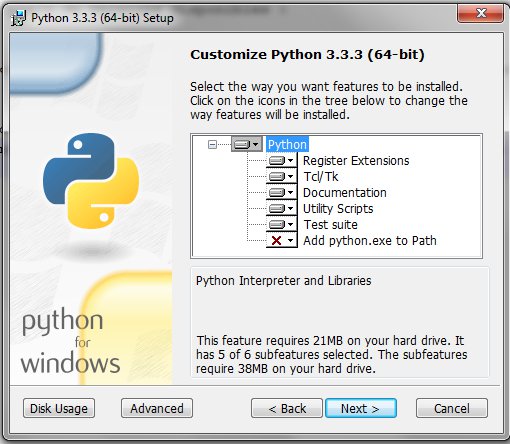
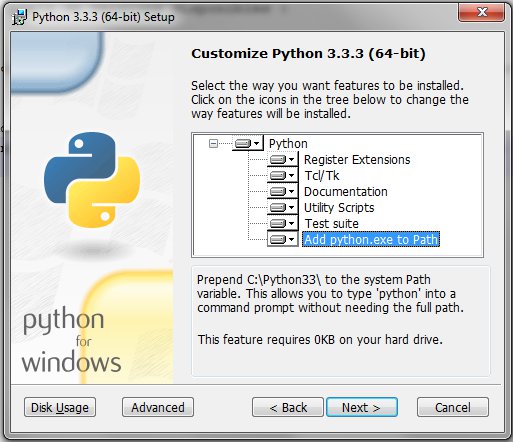
- Puis plus rien à faire, juste attendre un peu et cliquer sur
Finish
.
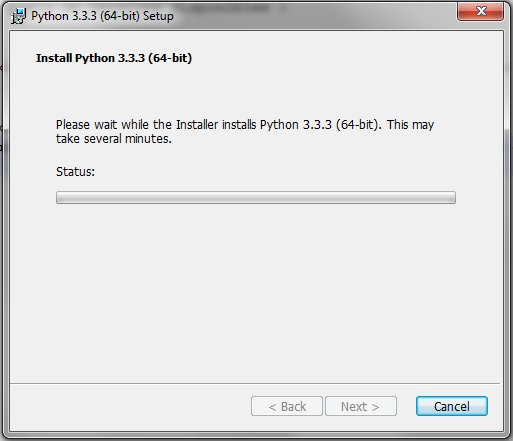
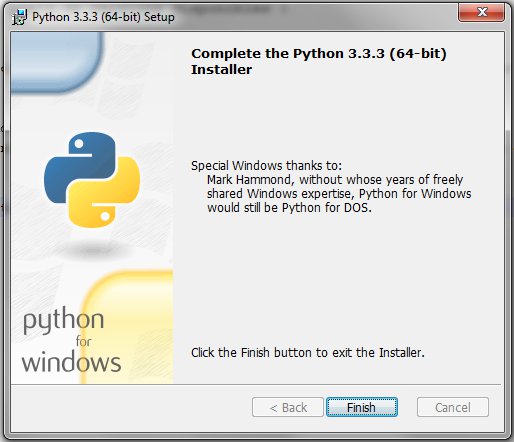
2. Utiliser la console Python
Une fois Python installé, la première chose est de tester que ça fonctionne !
Aller dans le menu démarrer, chercher et cliquer sur Python (command line)
.
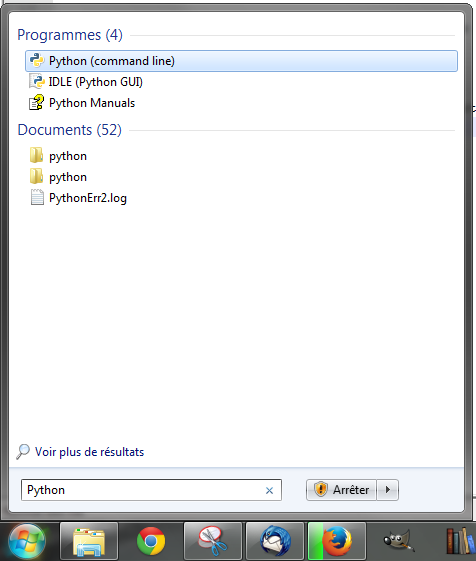
La console Python démarre : la première ligne affiche la version de Python installée (donc ici Python 3.3.3, version 64 bits).
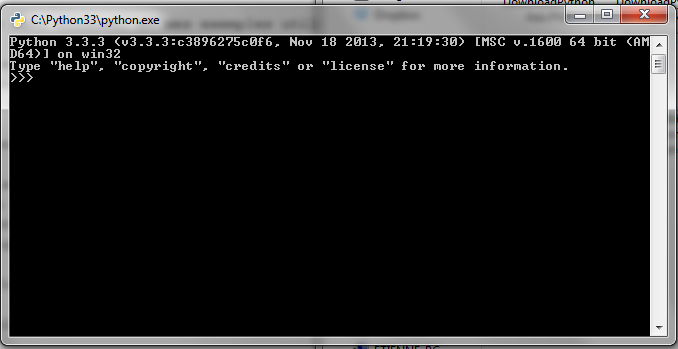
Tester une première commande, selon la tradition des tutoriaux de programmation, nous allons saluer le monde (mais en français), et plus précisément le monde de Python : taper print("Bonjour, Python !")
.
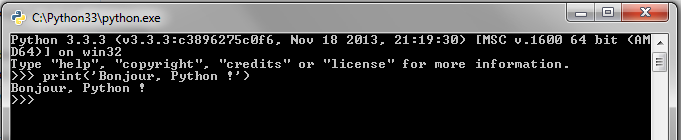
Bravo, vous avez installé Python avec succès ! Nous allons pouvoir passer aux choses sérieuses et apprendre à programmer !
Pour jouer avec :
- Remplacez les guillemets doubles par des guillemets simples : c’est pareil !
- Ecrivez une autre phrase.
- Essayez d’enlever les guillemets :
print(Bonjour, Python !)
: cela fait une erreur au niveau du point d’exclamation : SyntaxError: invalid syntax
. Et si on enlève le point d’exclamation ? Une autre erreur : NameError: 'Bonjour' is not defined
. On pourrait expliquer dans les détaills mais pour commencer, il faut retenir que si on ne met pas les guillemets, Python cherche à interpréter autrement les caractères que l’on écrit.
- Essayez en commençant avec un guillemet double et en terminant avec un guillemet simple, que se passe-t-il ?
- Essayez avec des caractères spéciaux
- Essayez en écrivant ‘Bonjour,\nmonde’, ‘Bonjour\tmonde’ : à quoi servent ‘\n’ et ‘\t’ ?
Pour comprendre : quelques explications.
print
est une fonction standard de Python, qui prend une chaîne de caractères et l’affiche dans la console. Une chaîne de caractères en Python est signalée par des guillemets simples (touche 4 '
) ou doubles (touche 3 "
).
On appelle caractère tout ce qu’on utilise quand on écrit : les lettres (abcdefghijklmnop…), les chiffres (0123456789), les signes de ponctuation (,?:!;), les symboles mathématiques (+-*/), par exemple, mais il en existe beaucoup plus (%ùàç€&$…). Si vous êtes curieux, vous pouvez lire l’article de Wikipédia sur les caractères informatiques.
La plupart du temps, on n’utilise pas un caractère tout seul, mais plutôt un mot ou une phrase. Pour dire à Python qu’on veut un mot ou une phrase, c’est-à-dire une “chaîne de caractères”, on les met ensemble entre guillemets, comme dans notre exemple : “Bonjour, Python !”. L’interpréteur Python sait alors que cette chose qu’on lui fait lire est une chaîne de caractères, et pas autre chose.
3. Un premier script
Pour écrire des scripts de façon très simple, il y a un éditeur qui est fourni avec l’installeur de Python : IDLE (Python GUI).
- GUI, ça veut dire quoi ? C’est de l’anglais pour “Graphical User Interface”, en français on dit une “interface graphique”. En fait, quand on a pas d’interface graphique, ça veut dire qu’on est dans une console. Sous Windows, vous utilisez l’écrasante majorité des logiciels avec une interface graphique.
Créez un répertoire sur votre ordinateur dans lequel vous allez mettre tous les fichiers liés à ce tutoriel (par exemple dans Mes Documents, un répertoire “IntroPython”).
Dans la fenêtre ‘Python 3.3.3 Shell’, cliquer sur File->New File, cela ouvre une autre fenêtre dans laquelle vous allez pouvoir écrire votre premier script. Tapez : print(“Bonjour, monde !”), puis sauvegardez le fichier en sélectionnant bien votre répertoire de travail (“IntroPython”).
Il faut maintenant exécuter le script. Pour cela, dans le menu de l’éditeur, cliquez sur ‘Run’, puis ‘Run Module’. Ou alors, encore plus simple, appuyez sur la touche F5. Voilà ce qui doit s’afficher dans la fenêtre “Shell” :
>>> ================================ RESTART ================================
>>>
Bonjour, monde !
On va essayer de faire un peu mieux. Effacez la ligne déjà écrites et copiez à la place les lignes suivantes :
print("Bonjour, comment t'appelles-tu ?")
chaine1 = input()
print(chaine1, ", quel joli nom !", sep='')
Pour comprendre :
print
est une fonction python, jusqu’à présent on lui passait en argument juste un chaîne de caractères, mais ici, à la troisième ligne, on voit qu’on peut lui en passer plusieurs. Chaque chaîne passée est affichée, en les séparants par la chaîne indiquée par sep=''
, ici, une chaîne vide, donc les chaînes sont collées. input
est aussi une fonction, et comme elle ne prend pas de paramètres, on l’appelle avec des parenthèses vides : input()
.
chaine1
est une variable, elle permet d’enregistrer une valeur et de la réutiliser plus loin. Ici, on l’initialise avec ce que nous renvoie la fonction input
, c’est-à-dire une chaîne entrée par l’utilisateur dans la console. Ensuite, on l’utilise pour afficher cette chaîne.
4. Un premier jeu
A partir du premier script, il est très facile de faire un quizz. Posez une question, vérifiez si la réponse est bonne, et comptez les points.
Par exemple :
"""
Un jeu de quizz
Le programme pose des questions,
l'utilisateur répond dans la console,
le programme compare sa réponse avec la bonne réponse
le programme ajoute 1 point par bonne réponse.
"""
# On commence avec aucun point
points = 0
# Question 1
# Affiche la question
print("1. Quelle est la capitale de la France ?")
# Récupère la réponse entrée par l'utilisateur dans une variable appelée capitale
capitale = input()
# Compare la réponse de l'utilisateur avec la bonne réponse, "Paris"
if capitale == "Paris":
# Donne un point si la réponse est bonne
points = points + 1
print("Bravo, bonne réponse !")
else:
print("Erreur ! La bonne réponse est : Paris.")
if points > 1:
print("Score :", points, "points")
else:
print("Score :", points, "point")
Pour comprendre
On apprend plusieurs choses avec ce tout petit bout de programme.
- En Python, il y a deux façons de faire des commentaires dans son code :
- pour faire un commentaire sur plusieurs lignes, on utilise des triples guillemets
"""
pour indiquer le début et la fin d’un commentaire,
- pour faire un commentaire sur une seule ligne, il suffit de commencer la ligne par le symbole dièse :
#
(touches Alt Gr
+ 3
).
- On utilise deux variables :
- une variable pour stocker la réponse donnée par l’utilisateur. Ici, on l’appelle
capitale
. On aurait pu l’appeler reponse1
. Ce qui est important, c’est de donner un nom qui permet de comprendre à quoi sert cette variable. Comme on y met une chaine de caractères (la réponse de l’utilisateur entrée dans la console), cette variable est de type “chaîne”.
- une variable pour stocker le nombre de points accumulés par le joueur, autrement dit, son score. Au départ, c’est zéro, et on l’augmente de 1 point à chaque fois qu’il répond bien. Les scores possibles sont donc 0, 1, 2, … mais jamais 0,5 ou 1,75. Notre score est forcément un nombre entier. La variable
points
est donc de type entier, en anglais on dit integer, et en programmation on écrit souvent int
.
- On fait un test avec le mot-clé
if
, en écrivant une “condition”, et en terminant le test par un double point :
.
- Les lignes à exécuter dans le cas où la condition est vraie sont écrites sur les lignes suivantes, avec une tabulation. Cette tabulation est importante, et c’est une des caractéristiques de Python. Vous pouvez utiliser des tabulations ou des espaces, mais vous devez respecter toujours le même alignement. Si vous vous trompez d’un espace, vous aurez une erreur (essayez !). On parle aussi d’indentation
- Ici la condition est une comparaison avec
==
: Python va comparer le contenu de capitale
avec la chaîne "Paris"
, et renvoyer True
si capitale contient exactement la chaîne "Paris"
(il ne faut pas se tromper sur la majuscule !).
- Si la condition est fausse (elle vaut
False
), c’est ce qui est après else:
qui est exécuté (toujours avec la nécessité de bien aligner les lignes d’instructions).
- On peut changer la valeur de la variable
points
très facilement : il suffit d’écrire points = <autre chose>
- en écrivant
points = points + 1
, on prend la valeur de point
(0
, dans notre programme), on y ajoute 1, et on met cette nouvelle valeur dans point
.
Pour jouer avec
Que se passe-t-il si au lieu de répondre exactement “Paris”, quelqu’un répond, “PARIS” ? Est-ce que c’est faux ? Non, il a juste utilisé des majuscules. Il connaît la capitale de la France. Mais notre programme lui dit qu’il se trompe. Pour changer ça, il faudrait pouvoir faire en sorte de comparer les lettres sans tenir compte du fait que ce sont des majuscules ou des minuscules. Une idée ? Une façon de faire ça, c’est de convertir capitale
en majuscules avant de faire la comparaison :
if capitale.upper() == "PARIS":
# Donne un point si la réponse est bonne
points = points + 1
print("Bravo, bonne réponse !")
else:
print("Erreur ! La bonne réponse est : Paris.")
Pratique : la fonction upper
, appliquée à une chaîne de caractères, la convertit tout en majuscules ! Et pareil, il existe lower
qui convertit tout en minuscules.
Mais, si quelqu’un est un peu bavard et répond : “C’est Paris !”, notre programme va encore lui dire qu’il se trompe. Comment faire ? Deux solutions : soit on précise à l’utilisateur qu’on ne veut que la réponse, et pas une phrase (on précise la consigne), soit on modifie notre programme pour qu’il comprenne la réponse. S’il trouve le mot “Paris” dans la réponse, c’est bon ! Python nous permet d’exprimer très facilement cette condition :
if "PARIS" in capitale.upper():
# Donne un point si la réponse est bonne
points = points + 1
print("Bravo, bonne réponse !")
else:
print("Erreur ! La bonne réponse est : Paris.")
On peut presque lire en traduisant littéralement : Si "PARIS"
est dans capitale.upper()
, alors…
Et si je teste avec une réponse de bavard :
>>>
1. Quelle est la capitale de la France ?
c'est paris
Bravo, bonne réponse !
Score : 1 point
Il est facile d’ajouter de nouvelles questions, à vous d’être inventif ! Faites attention à bien compter les points, vérifiez en affichant le score après chaque réponse.
Pour aller plus loin
Pour ajouter des nouvelles questions, on peut copier-coller le code et changer les questions. Très vite, le code va être très très long, alors que, finalement, on fait toujours la même chose : afficher une question, récupérer la réponse, comparer avec la vraie réponse, compter les points.
Un des grands principes en programmation, c’est de ne pas se répéter. En anglais, don’t repeat yourself, c’est-à-dire : DRY.
Comment faire ici ?
D’abord on voudrait pouvoir représenter une paire question-réponse. Une paire, c’est une liste de longueur fixe égale à 2. En Python, on peut représenter ça par ce qu’on appelle un tuple : un certain nombre de valeurs, séparées par des virgules, forment un tuple. On peut mettre des parenthèses autour pour mettre en évidence le fait que ces objets vont ensemble. Par exemple :
"Quelle est la capitale de la France ?", "Paris"
peut aussi se noter :
("Quelle est la capitale de la France ?", "Paris")
On peut maintenant représenter une paire question-réponse, mais dans un quizz, on voudrait en avoir plusieurs. Nous allons donc utiliser une autre structure de Python, la liste. Une liste regroupe un ensemble de variables qui ont le même type. Nous reviendrons plus tard sur ce qu’on peut faire avec des listes. Pour l’instant, nous allons juste faire une liste de questions-réponses :
question_reponses = [("Quelle est la capitale de la France ?", "Paris"),
("Quelle est la monnaie du Japon ?", "Yen"),
("Dans quelle ville ont eu lieu les Jeux Olympiques d'été en 2012 ?", "Londres"),
]
Une fois qu’on a cette liste de questions, on veut exécuter notre programme pour chaque paire de questions réponses. Pour afficher la liste des questions et des réponses, on peut faire :
for question, vraie_reponse in question_reponses:
# Affiche la question
print(question)
# Affiche la réponse
print(vraie_reponse)
Et voilà, vous avez fait votre première boucle for
! Comme on sait que notre liste contient des tuples (des paires), on peut récupérer directement chaque élément sous forme de paire question, vraie_reponse
(on crée directement deux variables).
Une “boucle”, comme son nom l’indique, consiste à répéter les mêmes instructions un certain nombre de fois (en boucle). Ici, avec la boucle for
(pour, en français), on répète les instructions pour chaque élément de la liste question_reponses
. Le double point :
, et l’indentation, c’est exactement comme avec le test if
.
On peut maintenant réécrire le programme pour qu’il pose plus de questions. C’est encore plus facile de poser des questions !
Encore quelques petites améliorations :
- pour faire plus joli, j’ai ajouté une numérotation. La fonction
enumerate
permet de renvoyer l’indice de la position de chaque élément de la liste, en plus de l’élément…
- au lieu d’écrire
points = points + 1
, ce qui est bien trop long, on peut écrire points += 1
:-).
Nous avons donc pour terminer le script suivant :
"""
Un jeu de quizz
Le programme pose des questions,
l'utilisateur répond dans la console,
le programme compare sa réponse avec la bonne réponse
le programme ajoute 1 point par bonne réponse
"""
# On commence avec aucun point
points = 0
question_reponses = [("Quelle est la capitale de la France ?", "Paris"),
("Quelle est la monnaie du Japon ?", "Yen"),
("Dans quelle ville ont eu lieu les Jeux Olympiques d'été en 2012 ?", "Londres"),
]
for i, (question, vraie_reponse) in enumerate(question_reponses):
# Affiche la question
print(i + 1, "-", question)
# Récupère la réponse entrée par l'utilisateur dans une variable appelée capitale
reponse = input()
# Compare la réponse de l'utilisateur avec la bonne réponse, "Paris"
if vraie_reponse.upper() in reponse.upper():
# Donne un point si la réponse est bonne
points += 1
print("Bravo, bonne réponse !")
else:
print("Erreur ! La bonne réponse est :", vraie_reponse)
if points > 1:
print("Score :", points, "points")
else:
print("Score :", points, "point")
You can download it here.